How to Design Climate-Forward AI Companies
There’s no denying that AI is having a moment right now. In 2023, more than one in four VC dollars spent went to AI companies, and that number seems poised to increase as investors look to this burgeoning technology to solve all kinds of human problems in innovative ways.
And while AI promises to provide major benefits to humanity—such as in predicting extreme weather events to save lives and protect property, and diagnosing ailments that would otherwise take years and multiple specialists to decipher—many founders, investors, and designers are not paying attention to another emerging concern: AI’s impact on the environment.
In the rush to innovate with AI, as a society we need to focus on more than just creating smarter machines and advancing technology—we also need to consider how we can build a more sustainable future. Recently, venture capitalist Daniel Goldman wrote an article about how investors can help every company become a climate company. As design-centric investors, we believe design has an important role to play in helping every company become a climate company, especially AI companies.
In this article, we’ll share why every AI company should be a climate company—and how thoughtful design can help us get there.
What if my AI company isn’t focused on the environment but still makes the world a better place?
You might be asking: If we’re building AI to help people work more productively and otherwise prosper, aren’t we doing enough good in the world?
The answer is, unfortunately: no.
In some ways, we live in a more complicated time for founders and corporations. Employees and customers alike care about environmental, social, and corporate governance (ESG) and demand a higher level of accountability from entrepreneurs and executives than in previous decades. This interest is starting to become law, too. With upcoming EU requirements on reporting Scope 1, 2, and 3 emissions, all companies will be held more accountable for their emissions.
Fortunately, studies that have tracked corporate data for decades show that companies with organizational processes to measure, manage, and communicate their performance related to ESG goals outperform their peers in terms of financial gains and higher valued stocks. They also have lower likelihoods of having large stock price declines or going bankrupt. Investors now understand that purpose does not divert from profitability.
At an AI x Climate webinar hosted by NationSwell in November, panelists shared why it’s important to focus on the climate impact of AI. Even if the tech sector itself is currently responsible for less than 4% of GHG emissions, if we continue with business as usual and do nothing to decarbonize the tech sector, by 2040 it will be responsible for 14% of global emissions.
That is why we should focus on growth areas, and AI is growing. The easiest time we will have to decarbonize AI is now.Asim Hussain, Chairperson and Executive Director of the Green Software Foundation (NationSwell, 2023)
The serious environmental costs of AI
Before getting into how design can help AI companies be more climate-friendly, it’s important to understand the full impact of AI-enabled technology on the environment. There are multiple costs that the average consumer may not be aware of.
To start, running AI is incredibly resource-intensive. And over the last decade, exponential growth in AI has driven capacity increases for AI training and inference (e.g. the work ChatGPT does to answer a new prompt), resulting in increased infrastructure and power capacity requirements.
For example, Forbes reports that AI tools fueled a 34% spike in Microsoft’s water consumption. In July 2022, the month before OpenAI says it completed its training of GPT-4, Microsoft pumped in about 11.5 million gallons of water to its cluster of Iowa data centers. Essentially, ChatGPT gulps up a 16-ounce water bottle every time you ask it as few as five questions.
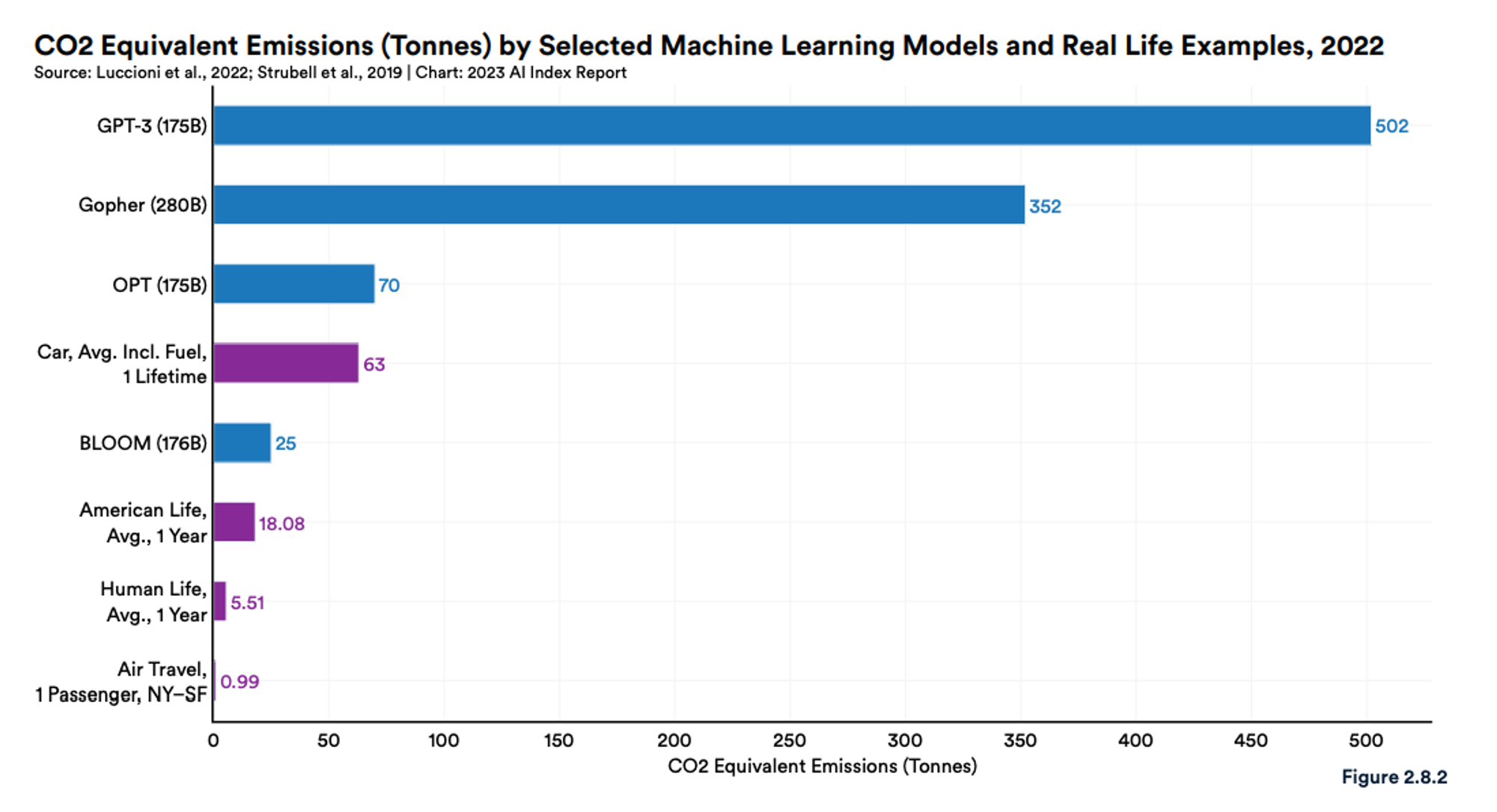
As we look to the future, another factor to keep in mind here is the Jevons Paradox—the economic principle that as costs of a raw material, good or service goes down, its demand and thus its resource use, goes up. For example, with efficiency gains and lower costs of power, overall power usage typically increases. In short, as AI becomes more energy efficient and inexpensive to access, its increased usage will accelerate AI’s effect on our planet.
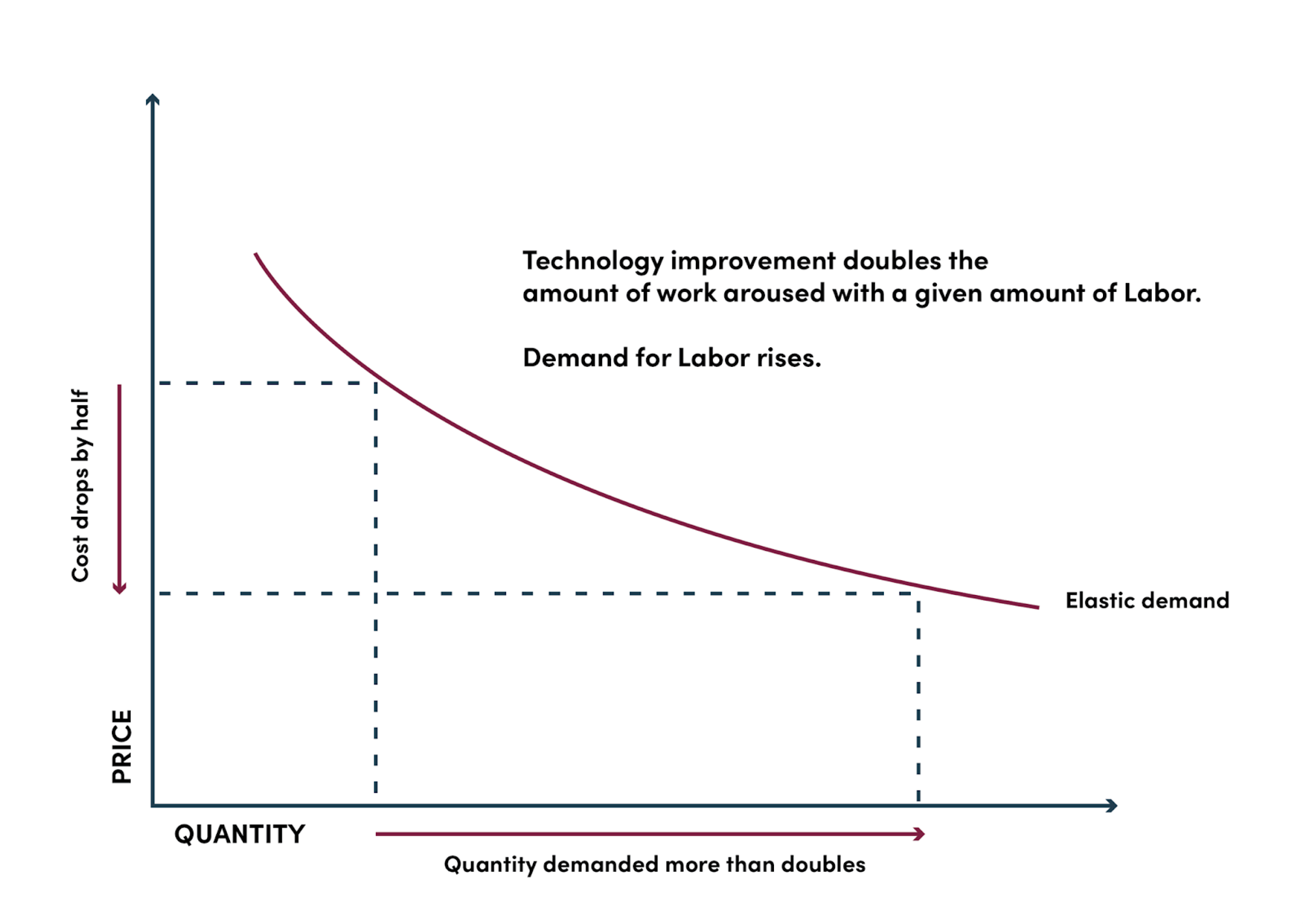
On top of the heavy resource consumption, computing also creates a lot of e-waste. The hardware needed to support AI contributes to its material footprint, including the mining and refinement of metals and rare earth elements used in batteries and semiconductor components. Less than 20% of the 50 million tons of e-waste. produced actually reaches formal recycling and waste management centers. The majority is illegally disposed of, polluting soil and waterways, as well as becoming a health hazard to the informal handlers of e-waste.
There is also little to stop the use of AI to further grow the fossil fuel industry. The Scientific American pointed out that Microsoft’s Azure would be used to optimize mining operations for ExxonMobil, increasing oil production by 50,000 oil-equivalent barrels per day by 2025.
Unfortunately, even the use of AI-enabled systems that have been designed to mitigate climate change raises serious challenges. Researchers at Oxford concluded that even when used for socially good purposes, AI-enabled systems risk undermining individual and group rights, self-determination, and foundational values of our societies, on top of having a large carbon footprint from GHG emissions.
The researchers proposed 14 policy recommendations to ensure more equitable and sustainable development of AI, including incentives for energy-efficient hardware and transparency in the carbon footprint of popular models to inform users about their environmental costs.
All of this brings up the question of impact: How might we design the systems around the usage and development of AI to have less—or even positive—environmental impact?
Opportunities for more efficient AI through design
As we move forward into the age of AI, new AI companies and their service providers need to think about how they can innovate on more sustainable terms. Design will play a key role by introducing thoughtful new approaches that consider the environmental, ethical, and social impacts of business decisions. Here are some of the emerging approaches.
I think AI companies should also be climate companies in terms of equitability and social impact. That’s a unique opportunity.Kamini Iyer, Design Lead at Lumen Energy
Designing for AI decarbonization
The geography of where model training takes place matters. For example, due to the required cooling demands for data centers, training on clusters in Iowa uses less resources than in Arizona for the same computing output.
Cowls et al. summarized how GHG emissions can vary across different countries due to the differences in energy sources (renewable or not) used by their electrical grids. A single training run of GPT-3 contributes over ten times the CO2eq emissions if performed in South Africa compared to in France.
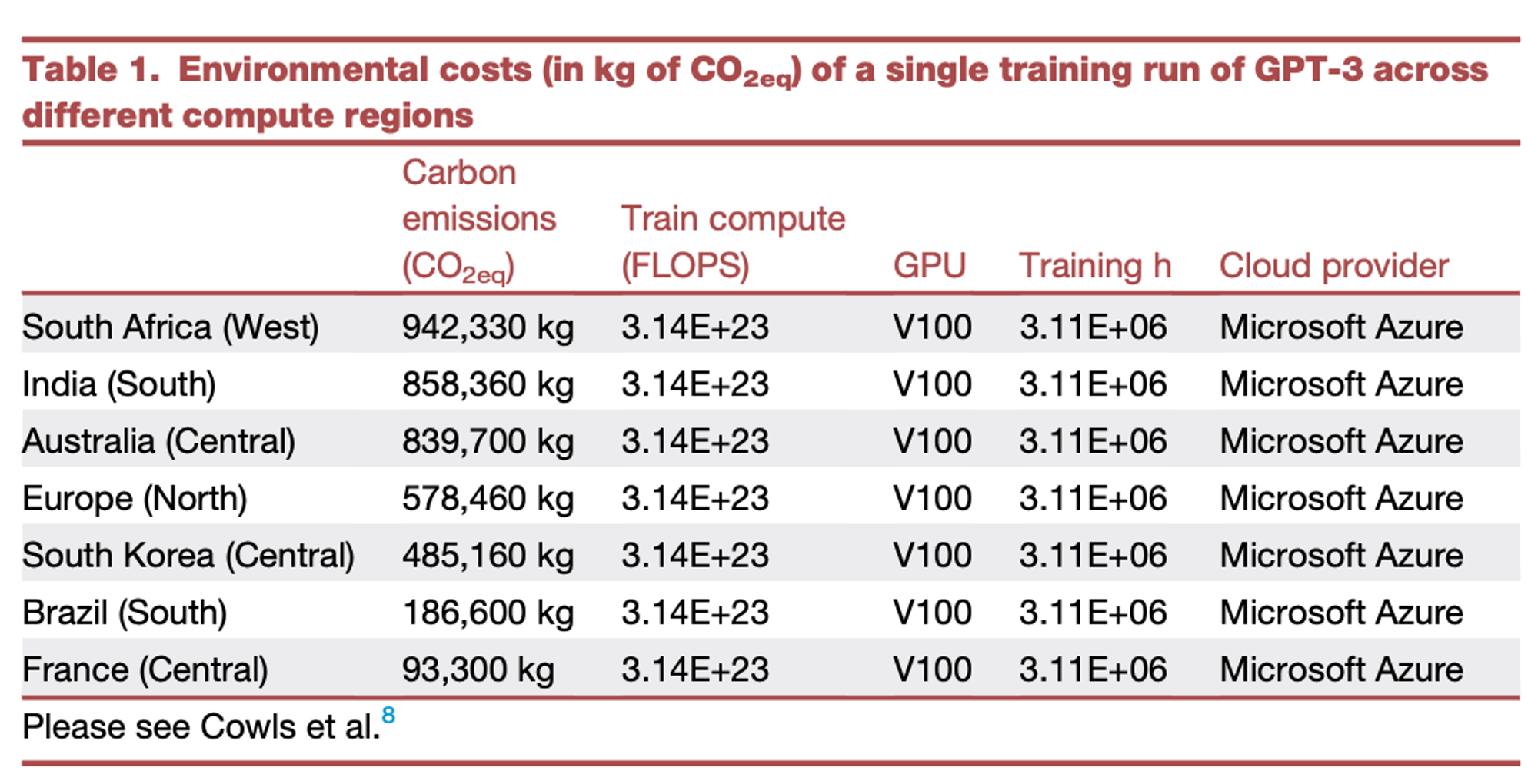
It’s easy to decarbonize AI training by considering the location of data centers and running models off renewable energy. However, the carbon footprint is harder to manage during inference, which is factors of magnitude larger—for example, when millions of customers summon AI chatbots from their cell phones and desktops. The costs of this power are trickled down across many users, leading to less focus on its environmental impact.
Luckily, inference can also easily be done on renewable resources. In order to decrease the overall carbon footprint of your company’s AI usage, business professors Ajay Kumar and Thomas Davenport offer some design and development decisions to consider:
- Use existing large generative models and fine-tune these models for training rather than developing your own.
- Employ energy-conserving computational methods. For example, running ML models on a local TinyML microprocessor instead of power hungry GPUs at data centers, uses 1000x less power.
- Be discerning about how and when AI is used. If a large model uses 3x the power but only improves your accuracy by 1-3%, it likely isn’t worth the extra energy consumption. Furthermore, evaluate your use case of generative AI—it is helping the world more than hurting it?
- Evaluate the energy sources of your cloud providers and data centers. Ideally, they run on 100% clean renewable energy such as hydroelectric and solar sources.
Designing for sustainable hardware and infrastructure
Hardware and infrastructure offer other opportunities for a design intervention. Efficiency is the name of the game here.
Some climate tech companies lower the carbon footprint of infrastructure that could be used for developing AI-enabled operations, like SeebeckCell Technologies, which transforms data center heat waste into electricity.
Other companies are exploring ways to more efficiently design within their existing infrastructure. Facebook AI (Meta AI) explored the environmental impact of AI by evaluating various machine learning model development cycles while also considering the life cycle of system hardware. Because companies such as Meta and OpenAI have customized data centers for machine learning tasks, they can optimize their computing needs for performance and power efficiency. With a Power Usage Effectiveness (PUE) of about 1.10, Meta’s data centers are nearly 40% more efficient than small-scale, typical data centers for cloud computing.
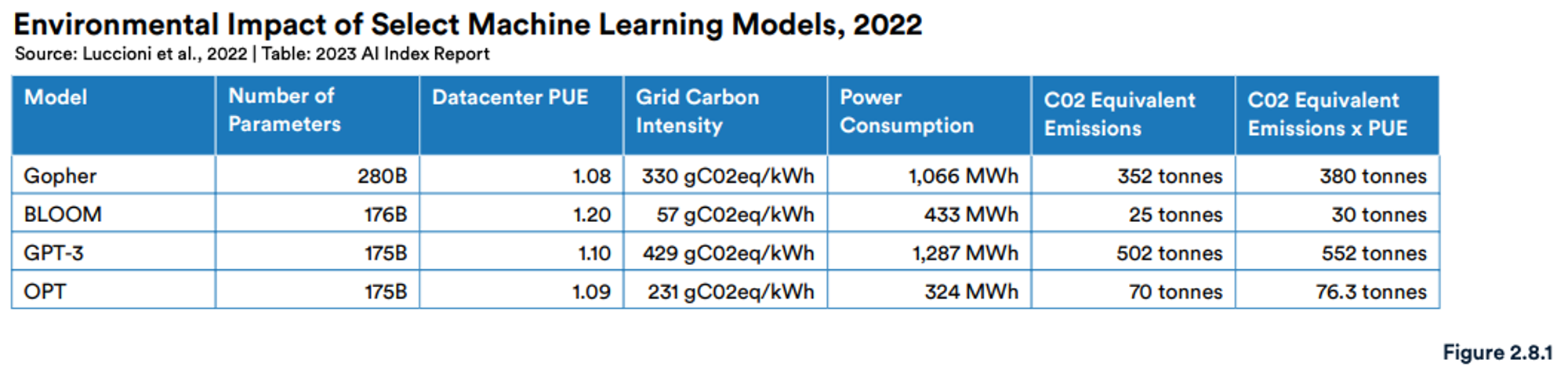
To further address the challenges of AI’s carbon footprint, Meta AI focuses on hardware-software co-design for efficient AI computing. This collaborative approach involves cross-layer optimization across the computing stack. Hardware coupled with software innovations for energy efficiency include:
- Using DRAM and Flash storage devices as caches, such that pre-computed embeddings can be shared across applications and use cases.
- Accelerated GPUs, specific for AI tasks.
- Algorithmic optimization such as reducing precision of operations (16-bit vs. 32-bit) and custom operators to schedule encoding steps within a single kernel (e.g. NVIDIA’s Faster Transformer.
Facebook AI achieved more than an 800x operational carbon footprint reduction through this approach for a Transformer-based universal language model. Overall power usage is reduced 20% every 6 months, however, accounting for the growth of AI use cases and the Jevons Paradox, the net effect is a 28.5% operational power footprint reduction over two years. By optimizing the design of AI systems, Meta AI aims to achieve competitive model accuracy while reducing computational and environmental costs.
DeepMind took a similar approach when it trained a reinforcement learning agent called BCOOLER (BVE-based COnstrained Optimization Learner with Ensemble Regularization) to optimize cooling systems at Google’s data centers. The results of the control algorithms demonstrated between 9-13% energy savings at two real world facilities. This type of hardware-software co-design can extend past data centers, and be applied to lower the carbon footprint of equipment for energy generation and storage, utility grids, and other preventative maintenance and operational applications.
The designer’s role in building sustainable and socially-forward AI companies
Beyond engineering and infrastructural design, there are a lot of opportunities for product designers, visual designers, communication designers, etc. to play a role in driving sustainable systems and influencing user behaviors. For example, by utilizing green design principles, UI/UX elements can also be designed for minimized energy consumption. Content loads (especially auto-loading) should be reduced; media and visuals can be minimized and simplified; and unnecessary data should be removed (e.g. minimize stored data and plan periodic data deletions). Project Drawdown’s Product Design Job Action Guide details more ways designers can execute on climate-related goals.
Translating complex concepts into digestible information
Kamini Iyer, Design Lead at Lumen Energy, is very familiar with how designers can respond to emerging technologies like AI from her work translating complex, dynamic green energy initiatives into streamlined user experiences. She shares how designers will need to shift their thought processes and skill sets as they start to integrate AI into their work.
The moment you allow an LLM or AI model to give you outputs, you’re now solving for the infinite—it has an infinite number of combinations and permutations it can spit out. This forces designers to think about data models and the biases that are in-built, something designers are not traditionally trained to think about when designing.Kamini Iyer, Design Lead at Lumen Energy
Given the surge of opportunities and outputs AI will unlock, Kamini believes designers will be challenged to think more like data scientists, while also wearing the designer’s hat and finding novel ways to use AI while building trust with users.
AI will require us to establish new habits, patterns, think about inclusivity, and develop more equitable solutions—things we think about when establishing design systems.Kamini Iyer, Design Lead at Lumen Energy
Designing the AI nutritional label
Policy makers can support innovations in sustainable AI by mandating more transparency around AI products’ carbon footprint. Designers can build on this transparency by offering new user interfaces to help digest such information. For example, prominently displaying information about how a product utilizes AI, such as specifying the model it was built with or outlining how customers' data will be used.
Jenn Huffstetler, Chief Product Sustainability Officer at Intel, explains how consumer behavior can be influenced by knowledge, akin to an AI nutritional label—a quick way to understand the true cost of your AI consumption.
Governments could put it into policy so that individuals can have an informed choice, because absent that standardization, consumers currently do not have tools to modulate their consumptionJenn Huffstetler, Chief Product Sustainability Officer at Intel (NationSwell, 2023)
Twilio created an AI Nutrition Facts generator to help designers convey transparency and accountability on how user’s data is being used. We’d like to see this type of work expanded to include environmental impact factors such as carbon footprint, energy usage, and water consumption data for AI products.
Developing—and living up to—AI codes of conduct
Beyond finding ways to communicate transparently with users, AI designers and entrepreneurs will need to explore, define, and apply ethical guidelines for the use of AI. These guidelines should consider both the human impact and the environmental impact of their work. Designers are in a key position to help distill, communicate, and codify these codes of conduct—ensuring that a company’s external-facing products live up to these values.
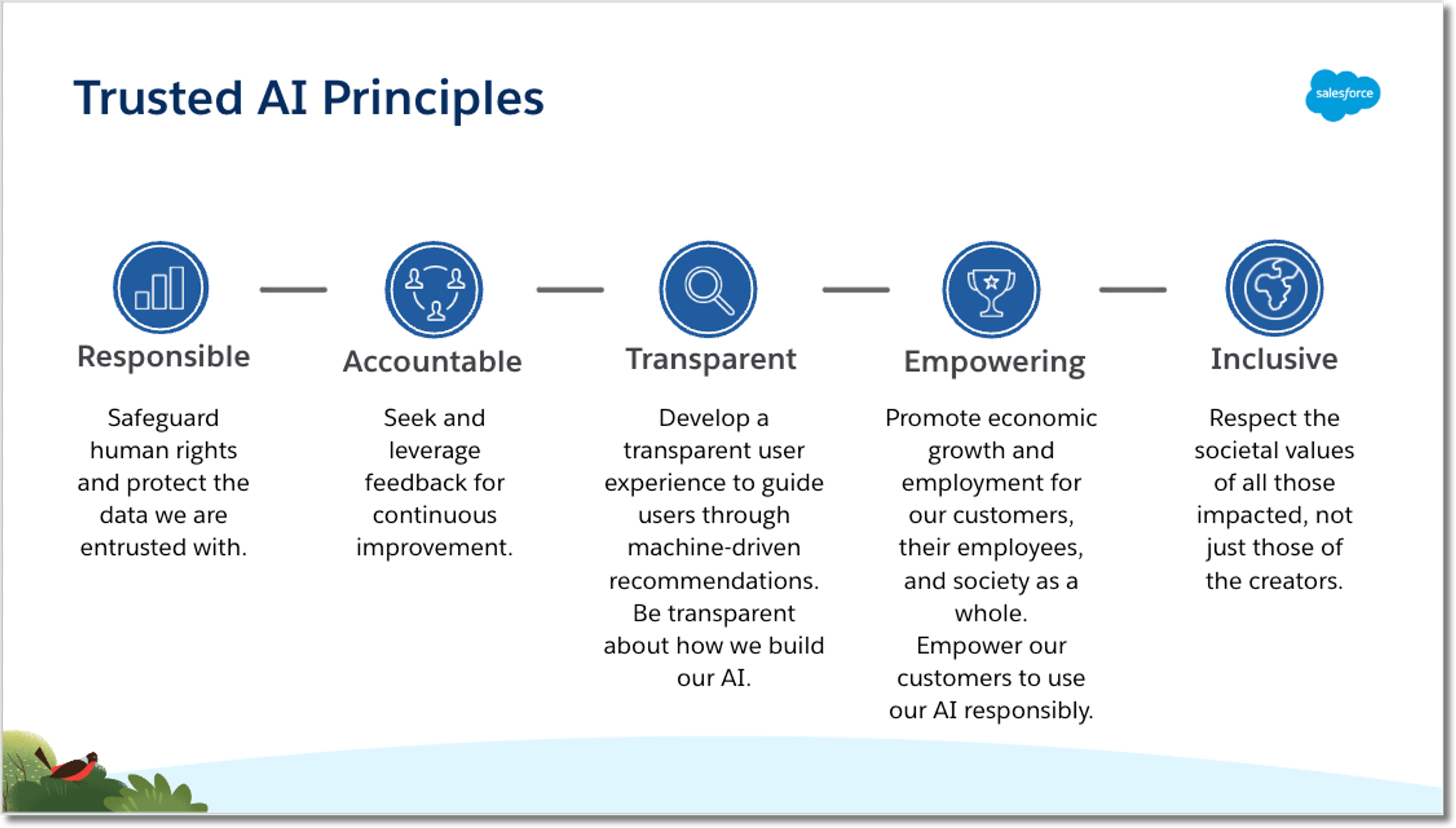
Codes of conduct can cover everything from bias and discrimination to electricity usage. Scott Gigante of Sightline Climate points to organizations such as G7 and Salesforce that have public codes of conduct for the use of AI. The EU is taking a first step towards enacting such guidelines into law with the newly adopted AI Act, in order to ensure AI systems used in the EU are safe, transparent, traceable, non-discriminatory and environmentally friendly. Similarly, President Biden’s Executive Order on Safe, Secure, and Trustworthy Artificial Intelligence can serve as a blueprint for internal codes of conduct to drive design decisions.
Founders have an opportunity to get these practices into the company’s framework as soon as possible, getting ahead of carbon accounting mandates, implementing policies of transparency, and building trust with customers today.
If you are in a leadership position in any organization that is developing or using artificial intelligence, I’d love to see you develop a code of conduct for your internal use of AIScott Gigante, Founding Engineer at Sightline Climate (NationSwell, 2023)
Climate-friendly actions for AI companies
While understanding the full impact of AI on the environment is a complex process, there are luckily a lot of things AI companies can do to start being more climate-conscious and socially forward today. Here’s an abbreviated checklist of tips and tools for founders and designers:
For founders:
- Reduce and recycle resources in all parts of your business, and don’t overlook Scope 3 emissions from your partner organizations.
- Use renewable energy sources whenever possible.
- Consider your computing location, which can greatly affect your operational carbon footprint.
- Practice carbon aware computing by deciding when and where to perform computing tasks. For example, AI training can be done during times of excess available clean energy.
-
Perform Life Cycle Assessments on your products and services, and share the results with your customers.
- For the transportation and mobility industry, the Greenhouse Gases, Regulated Emissions, and Energy Use in Transportation (GREET) model is a tool to examine the life-cycle impacts of vehicle technologies, fuels, products, and energy systems.
- For companies in the apparel and footwear industry, the Higg Index can be utilized to assess and measure the social and environmental performance of the value chain and the environmental impacts of products.
- Follow green software principles. Check out the Green Software Foundation, take a course on Green Software Principles, and get insights from sustainable software experts.
For designers:
- Check out Project Drawdown’s Product Design Job Action Guide for resources on how to align your everyday work outputs with green design principles, ESG goals, circular solutions and more.
- Learn more about how other designers are approaching AI. Read our article on how innovative companies are harnessing AI with design today, with insights from leaders at Figma, Midjourney, Databricks and Modyfi.
- Lean into your skills as a communicator—your work is crucial for connecting your company with customers, and helping everyone understand the impact of their AI decisions on the environment.
We truly believe that smart design choices can help all companies reduce AI’s impact on the environment, not just the climate-focused endeavors. We hope you keep some of these sustainability tips in mind while developing your AI-enabled company. Happy building!
Hero image created with Visual Electric, a Designer Fund portfolio company).
Dr. Santhi Analytis is a technologist based in the San Francisco Bay Area. She’s gone from building breast pumps to heat pumps, as the CTO & co-founder of Moxxly, Inc. (acquired by Medela) and the founding VP of Engineering at Gradient. She was the 2023 Venture Fellow and is currently a Venture Advisor at Designer Fund.